Architecture has greatly adopted many technological breakthroughs when compared to other fields. In the last decades, architectural practices have changed a lot due to computational changes.
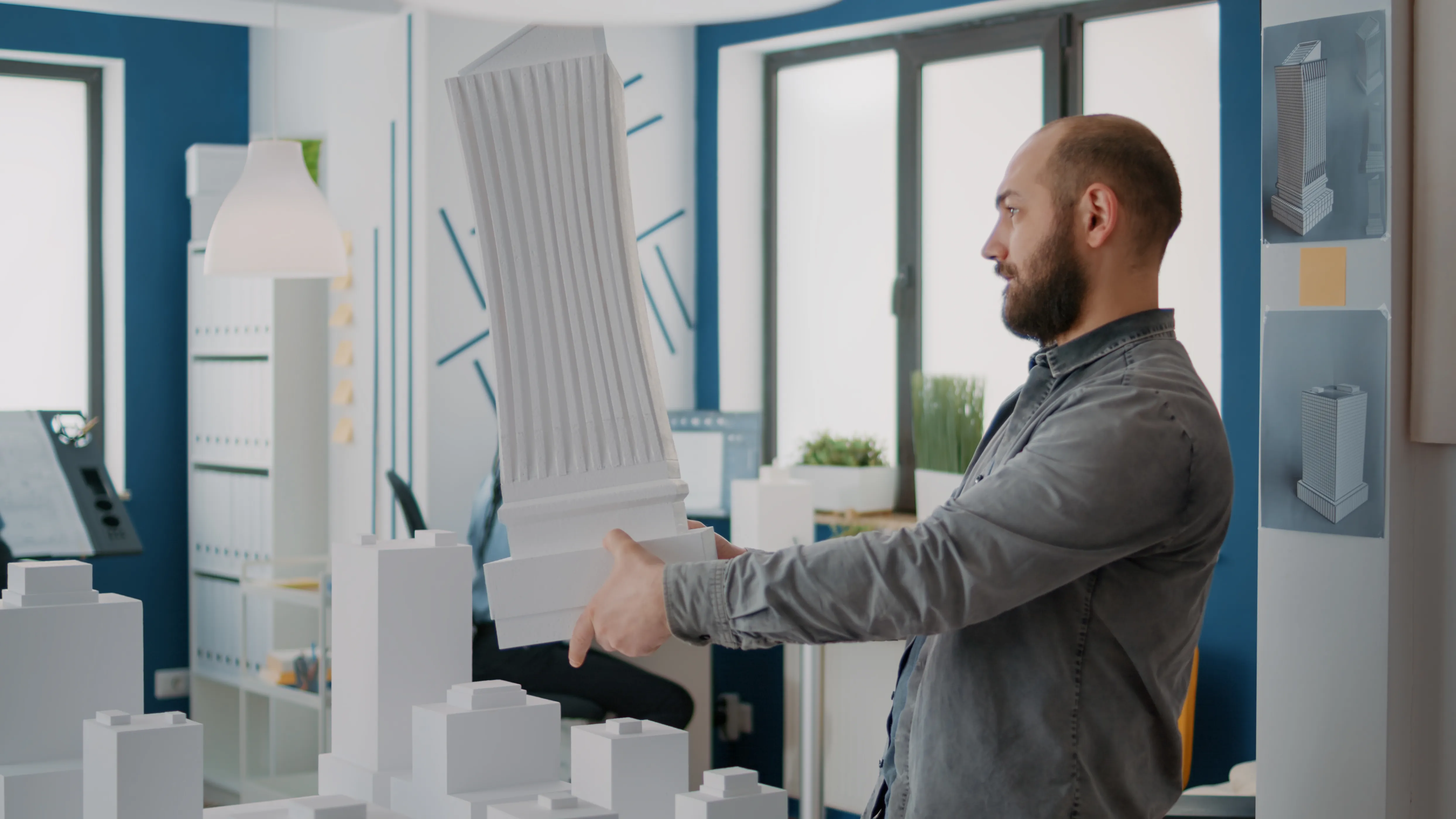
However, it is mandatory to understand the foundations of Machine learning (ML) to get a clear understanding of how this ML will impact this architectural practice and other fields in the future. ML techniques are successfully applied by companies like Tooliqa and AntWork
Let us discuss some hypothetical uses of ML in architectural designs and the ML approaches in conceptualization.
Introduction
The computational revolution changed the architectural designs and the way architects design, and these computational methods are completely embedded with the current methods of architecture.
In addition, the new computational revolution is driven by recent breakthroughs of ML in fields like medicine, physics, and finance.
ML is a subdivision of AI’s non-symbolic branch that deals with computational statistics and optimization procedures and uses self-improving learning methods to perform specific tasks.
ML builds computational systems which are not programmed to perform a specific task instead it builds a mathematical model using training data and adapts its parameters to enhance its performance on specific tasks without any human intervention.
Further, ML is used not only to automate tedious tasks but also is used for decision-making across miscellaneous domains. In architecture, the ML is used for optimized control strategies, urban planning, construction modelling but soon it is expected to have a large impact on architecture.
The educational framework for teaching ML to architects is to facilitate techniques in the deployment of design methods resulting in more instinctive design tools.
Moreover, AI created systems with human cognitive capabilities but was often limited by computational resources whereas ML systems achieved human-level performance and surpassed it by performing various complex tasks.
Concepts behind Machine Learning
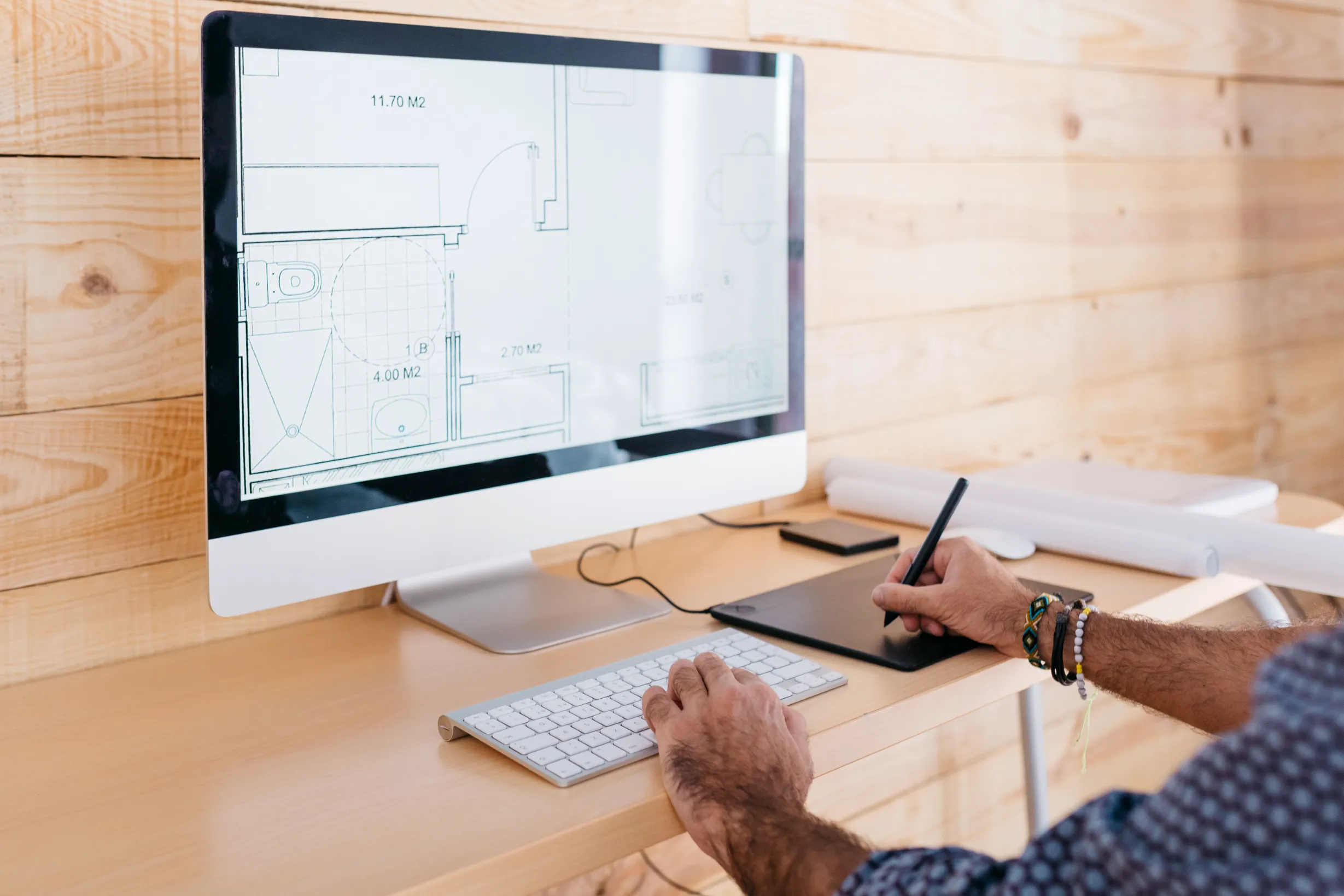
Machine learning is otherwise called statistical learning which uses algorithms to learn from the data. ML requires a training set of data that hold past experiences where the algorithm builds a mathematical model to make predictions using the training data.
In addition, a good ML system predicts accurately based on the given training set and the features associated with it. ML approach differs based on the problem and data.
The fundamental ML methods are
Supervised learning
A training set holds both inputs as well as output. They are also called associated labels. In supervised learning, the output is used to guide the learning process for classification, regression, and ranking-related problems.
Unsupervised learning
A training set holds only input and is also called a labelled training data set. Unsupervised learning is often used to predict the data based on how they are organized, and this is mainly used to perform clustering and dimensionality reduction problems where it is difficult to evaluate the performance of unlabeled data sets.
The most common mathematical models used in ML are Artificial neural networks, Decision trees, K-means, Bayesian networks. Many ML techniques require a huge amount of data which is a problem regarding settings where getting a large amount of data is not that easy.
Moreover, to bridge this data gap using the transfer learning methodology which transfers the knowledge between the tasks by applying relevant knowledge from the previous experiences to enhance the performance with fewer data.
Architecture and design: The impact of machine learning
ML uses the learning algorithms that resemble frequently applied reasoning processes by the architect to enhance the design process such as abductive reasoning, which acts as logical inference.
Conceptualization
Conceptualization is carried out at earlier design styles that hold main ideas and strategies. The conceptualization tasks are carried out in two different approaches namely-
(i) Bottom-up approach: Various ideas and strategies are created in an incrementation process to form a complex design.
(ii) Top-down approach: A high-level design is idealized and processed in different ways to create the corresponding design.
Artificial neural networks and deep learning
ML can identify the patterns and correlations in hidden data to enhance the conceptual design process. This helps in retrieving various conceptual ideas using a pre-defined vocabulary based on the client’s requirements.
ML retrieves the concept either in textual or visual pattern and rapidly explores design concepts which in turn reduces the time of researching design references.
Further, in textual representations, ML techniques use natural language processing tasks, such as automatic, text simplification, etc. By using the input, it is possible to encode them into design features.
In addition, there is a simplified method in which the information is represented using vocabulary and algorithms to learn associations between how input is related to output.
In visual representation, uses the textual outputs of the previous experiences to produce equivalent visual designs. This technique is known as text-to-image synthesis where characters are translated to pixels.
ML translates the text-to-3D models where the difficulty lies to produce accurate 3D models based on the features.
Recommender systems in ML
A recommender system provides suggestions that help users to make decisions. Moreover, a recommender system enhances the conceptualization tasks by,
(i) Coding the existing pattern as templates.
(ii) Analyzing the interactions between the architect, his architecture and design tool to provide suggestions for the upcoming conceptual designs.
(iii) Learning from the frequently used operations to enhance architectural design tool
(iv) suggest architect which yields poor performance in terms of predefined criteria. Moreover, there are also potential risks in applying these approaches to overcome these problems, there should be a continuous update.
Style transfer
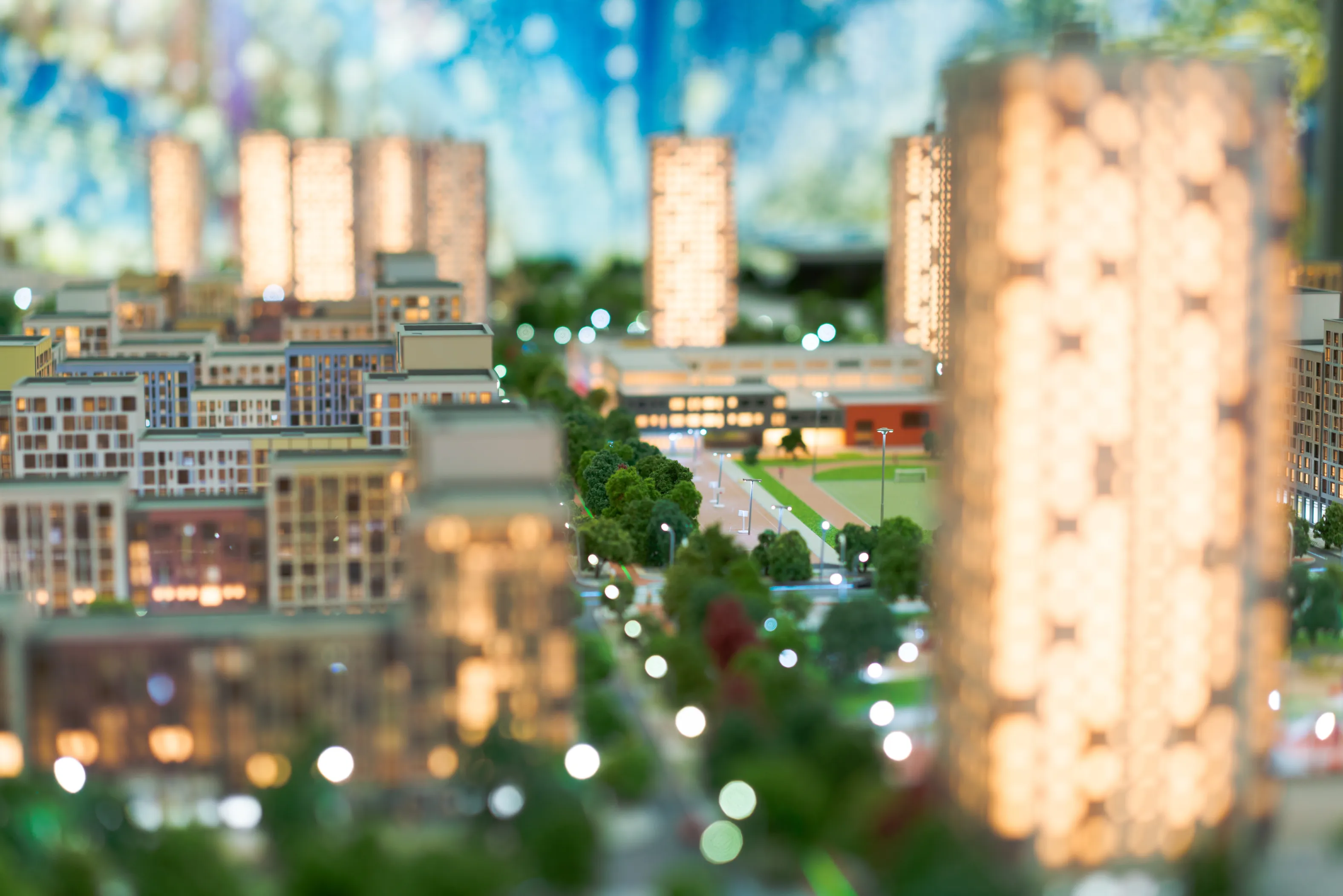
Style transfer applies different design styles to building and uses convolutional neural networks to obtain the style of an input image. This uses two different images and blends the style.
Moreover, the style transfer techniques aid in urban planning and mass customization problems. The style transfer techniques use two approaches:
- Uses the image sequence to produce designs.
- Uses geometrical properties to produce designs.
Image-to-design synthesis
Image-to-design synthesis uses neural style transfer which collects structures from the image to produce a design. Further, an ML model generates design from an image which is further incorporated with machine translation.
Clustering and dimensionality reduction
A model categorization in early design stages is considered to have a faster exploration of design space. Unsupervised learning techniques explore larger design space and groups in distinct categories based on the condition.
In the case of high-dimension design space, this technique reduces the dimensions for smoother visualization. Creating a geometry from a textual description based on the existing mechanism benefits the top-down approach.
Also read: Impact Of 3D Modelling on Architectural Design Education (tooli.qa)
Tooliqa specializes in AI, Computer Vision and Deep Technology to help businesses simplify and automate their processes with our strong team of experts across various domains.
Want to know more on how AI can result in business process improvement? Let our experts guide you.
Reach out to us at business@tooli.qa.