Generative AI is a type of artificial intelligence that is able to generate new and original content, such as text, images, or music. It has the potential to revolutionize many industries, from media and entertainment to advertising and design.
One of the most popular recent examples of generative AI is ChatGPT, which has become viral due to its capabilities. It is a chatbot that uses a variant of the GPT-3 language model to generate human-like responses to user input.
Other examples of generative AI include the use of GANs to generate realistic images of people, animals, and objects, such as the "This Person Does Not Exist" website which generates images of photorealistic human faces.
In the music industry, generative AI has been used to compose original pieces, such as Amper Music's AI-powered music composition platform.
In the fashion industry, generative AI has been used to design and produce original clothing items, such as the "AI-generated" fashion line produced by French luxury brand Balmain.
These techniques are driven by deep learning and other advanced AI algorithms, which allow them to learn and generate content that is increasingly sophisticated and realistic.
As we explore the techniques driving generative AI, including deep learning and GANs, we may ask ourselves: what other industries and applications could potentially benefit from the capabilities of generative AI?
In this blog, we will discuss the current capabilities and future potential of these techniques, and how they may shape the way we create and consume content in the future.
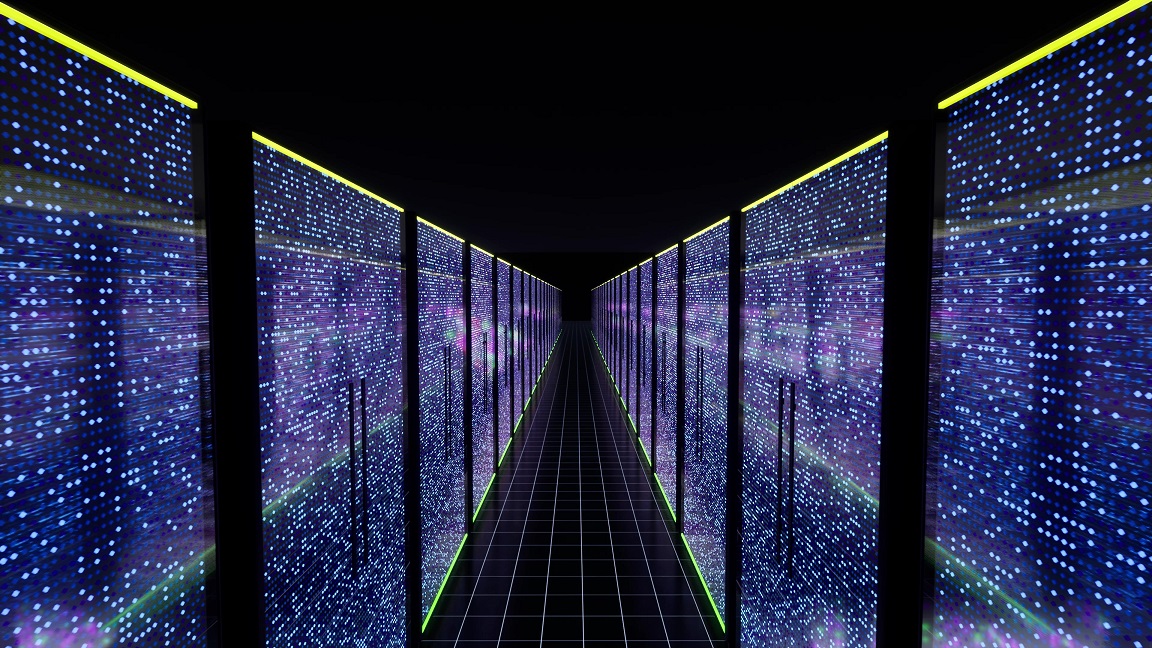
Deep Learning: An Overview and Its Role in Generative AI
Deep learning is a subfield of machine learning that involves the use of artificial neural networks to learn and make predictions based on data. These neural networks are composed of multiple layers of interconnected nodes, which process and transform input data in a hierarchical manner.
In the context of generative AI, deep learning algorithms are trained on large datasets of text, images, or other types of content.
During the training process, the algorithms analyze the data and learn the patterns and structures present in the data. This learning is achieved through the adjustment of weights and biases within the neural network, which are updated based on the error between the predicted output and the desired output.
One of the key advantages of deep learning in generative AI is its ability to handle a wide range of data types and formats, including text, images, and audio. It can also be used to generate content in multiple languages, making it a powerful tool for creating multilingual content.
Deep learning has been widely used in various generative AI applications, such as chatbots, image generation, and music composition. It has also been applied in the creation of personalized advertising content, by generating ads based on users' browsing history or interests.
As deep learning algorithms continue to improve and become more sophisticated, they are likely to play an increasingly important role in the development of generative AI.
Generative Adversarial Networks (GANs): An Introduction and Examples of Their Use
Generative adversarial networks (GANs) are a type of neural network that have been widely used in generative AI. They consist of two networks: a generator and a discriminator. The generator is trained to produce synthetic data, while the discriminator is trained to distinguish between synthetic and real data.
During training, the generator produces synthetic data, and the discriminator tries to distinguish it from real data. If the discriminator is successful, it provides feedback to the generator, which adjusts its parameters to produce more realistic synthetic data. This process continues until the discriminator is no longer able to distinguish between synthetic and real data.
One of the key advantages of GANs is their ability to generate high-quality, realistic images and other types of data. They have been used in a variety of generative AI applications, including the creation of photorealistic images of people, animals, and objects. GANs have also been used to generate other types of content, such as music and video.
For example, in the film and television industry, GANs have been used to generate realistic special effects and backgrounds, such as the use of GANs to create the digital environments in the movie "Blade Runner 2049."

Other Techniques in Generative AI: VAEs and Autoregressive Models
In addition to deep learning and GANs, there are several other techniques that have been used in generative AI. These include variational autoencoders (VAEs) and autoregressive models.
Variational Autoencoders (VAEs)
VAEs are a type of neural network that are trained to encode and decode data. They consist of two parts: an encoder and a decoder. The encoder maps the input data to a latent space, while the decoder maps the latent space back to the original data. During training, VAEs are trained to minimize the reconstruction error between the input data and the decoder's output.
VAEs have been used in a variety of generative AI applications, including the generation of images and audio content. For example, VAEs have been used to generate original music compositions and to synthesize realistic human speech.
Autoregressive Models
Autoregressive models are a type of generative model that generate data by predicting the next element in a sequence based on the previous elements. They have been widely used in natural language processing tasks, such as language translation and text generation.
For example, the GPT-3 language model is an autoregressive model that has been used to generate human-like text responses in chatbots and other applications.
Comparisons of Different Techniques in Generative AI and Their Trade-Offs
As we've seen, there are several techniques that have been used in generative AI, including deep learning, GANs, VAEs, and autoregressive models.
Each of these techniques has its own strengths and limitations and choosing the right technique for a given application may depend on the specific goals and constraints of the project.
For example, deep learning is a powerful technique that is able to learn and generate a wide range of content types and formats. However, it can be computationally intensive and may require a large amount of training data to achieve good results.
GANs, on the other hand, are able to generate high-quality, realistic images and other types of data, but may be more prone to mode collapse, where the generator produces a limited range of outputs.
VAEs are able to generate diverse content and can be trained on smaller datasets but may produce less realistic results compared to GANs.
Autoregressive models are able to generate sequential data, such as text, and can be trained on relatively small datasets, but may struggle to generate content that deviates significantly from the training data.
In addition to these trade-offs, there are also ethical considerations to keep in mind when using generative AI.
For example, the use of deep learning and GANs to generate synthetic images and other content raises questions about the potential for misuse, such as creating fake news or propaganda.
Therefore, when choosing a generative AI technique, it is important to consider the specific goals and constraints of the project, as well as the trade-offs and ethical implications of each technique. Careful consideration of these factors can help ensure that generative AI is used effectively and ethically in a variety of applications.

The Future Outlook for Generative AI Techniques
As generative AI techniques continue to improve and become more sophisticated, there are many potential applications and implications to consider. Here are a few possible examples of how these techniques could shape the future:
Personalization
Generative AI techniques could be used to create personalized content for users, such as personalized advertising, news articles, or social media posts. This could enable companies and organizations to better target their content and create more engaging experiences for users.
Automation
Generative AI techniques could be used to automate the creation of content, such as by generating news articles or social media posts based on templates or other inputs. This could save time and resources, while also enabling the creation of new types of content.
Creative expression
Generative AI techniques could be used to enhance or expand upon human creativity, such as by generating original music compositions or artwork. This could open up new avenues for creative expression and collaboration between humans and AI.
Information management
As generative AI techniques become more sophisticated, it is possible that they could be used to generate a vast amount of content. This could present new opportunities for users to find and access relevant information, as well as new challenges for managing and organizing the vast amounts of data generated by these techniques.
Are you ready to take your business to the next level with the power of AI? Look no further than Tooliqa!
Our team of experts is dedicated to helping businesses like yours simplify and automate their processes through the use of AI, computer vision, deep learning, and top-notch product design UX/UI.
We have the knowledge and experience to guide you in using these cutting-edge technologies to drive process improvement and increase efficiency.
Let us help you unlock the full potential of AI – reach out to us at business@tooli.qa and take the first step towards a brighter future for your company.