In the previous blog, we introduced the concept of data labeling and discussed the different types of data labeling, as well as tips for creating an efficient process.
Read the blog here: Efficient Data Labeling: Tips and Techniques for Machine Learning (tooli.qa)
In this blog, we will delve further into the decision of whether to use human annotators or machine learning algorithms for data labeling and weigh the pros and cons of each approach.
We will also explore the ethical considerations of data labeling, including best practices for ensuring data privacy and fairness.
Finally, we will examine real-world examples of how data labeling has been used to improve the accuracy of machine learning models and consider the future of data labeling in the age of artificial intelligence.
Human annotators versus machine learning algorithms
One key decision in the data labeling process is whether to use human annotators or machine learning algorithms to classify the data. Both approaches have their own advantages and disadvantages, and the best method will depend on the specific requirements of the task and the data set.
Human annotators have the ability to understand and classify data with a high level of nuance and complexity.
They can also identify patterns and trends that may not be immediately apparent to machine learning algorithms. However, human annotation can be time-consuming and labor-intensive, and it is prone to error and inconsistency.
Machine learning algorithms, on the other hand, can classify data much more quickly and efficiently than human annotators.
They can also handle large volumes of data with ease, making them well-suited for tasks with large data sets. However, machine learning algorithms may not be as accurate as human annotators, particularly for tasks with complex or nuanced labeling requirements. They may also struggle to identify patterns or trends that are not explicitly programmed into the algorithm.
Ultimately, the choice between human annotators and machine learning algorithms will depend on the specific needs and goals of the data labeling task.
It may be necessary to use a combination of both approaches in order to achieve the best results.
Ethical considerations of data labeling
In the era of machine learning and artificial intelligence, it is important to consider the ethical implications of data labeling. This includes issues related to data privacy and fairness in the labeling process.
To ensure data privacy, it is important to protect the personal and sensitive information of individuals included in the data set. This may involve implementing anonymization techniques or obtaining explicit consent from individuals before using their data.
In terms of fairness, it is important to ensure that the data labeling process does not perpetuate biases or discriminate against certain groups. This may involve considering diversity in the data set and making sure that the labels are applied consistently and objectively.
There are a number of best practices for ethical data labeling, including:
- Ensuring that data privacy is protected through anonymization techniques or explicit consent from individuals
- Evaluating the data set for potential biases and taking steps to address them
- Providing clear guidelines and training for annotators to ensure consistent and objective labeling
- Regularly reviewing and evaluating the data labeling process to ensure fairness and accuracy.
By following these best practices, you can help ensure that the data labeling process is ethical and responsible.
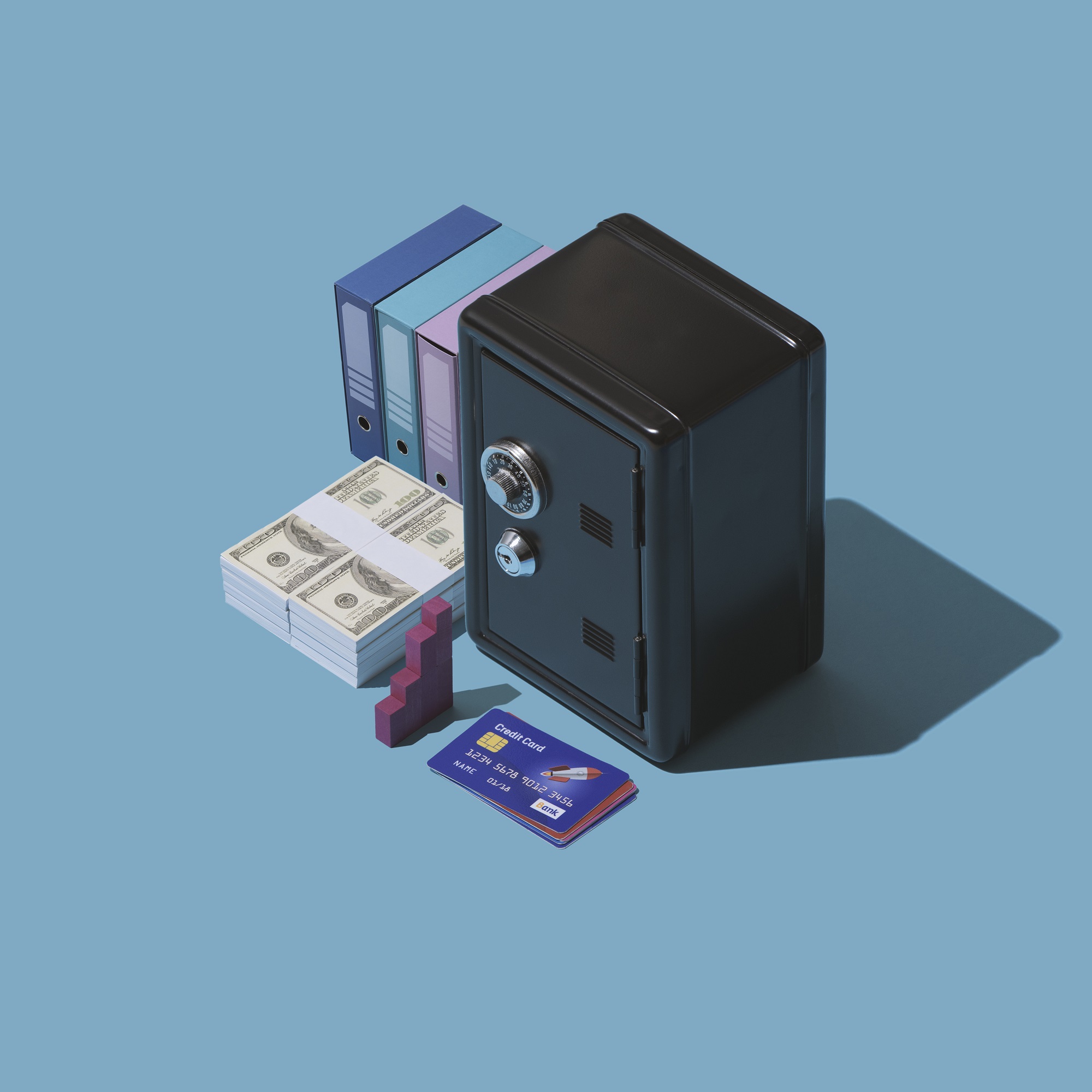
Real-World Impact: How Data Labeling is Improving the Accuracy of Machine Learning Models
Data labeling has been widely used in various applications of machine learning and AI, and many companies and organizations have seen improvements in their machine learning models as a result of accurate and efficient data labelling.
Image recognition
A company used data labeling to train a machine learning model to identify and classify objects in images with high accuracy. This technology is now widely used in applications such as self-driving cars and product recommendations.
Finance
Data labeling has been used to train machine learning models to detect fraudulent transactions and suspicious activity. Banks and financial institutions use these models to monitor large amounts of financial data and detect patterns that may indicate fraud.
Manufacturing
Data labeling has been used to train machine learning models to predict maintenance needs for industrial equipment. By analyzing sensor data from equipment and identifying patterns that indicate impending failures, these models help companies reduce downtime and improve efficiency.
Logistics and supply chain management
Data labeling has been used to train machine learning models to optimize delivery routes and predict demand for products. These models help companies reduce transportation costs and improve customer service.
Healthcare
Data labeling has been used to train machine learning models to assist in medical image analysis and diagnostics. This technology can help radiologists and medical practitioners identify and diagnose diseases, such as cancer, more efficiently.
Natural language processing
Data labeling has been used to train machine learning models to understand and analyze customer feedback, sentiment analysis, and dialogue generation. This technology is commonly used by companies in order to improve their customer service and gain insights from customer interactions with their products/services.
Future of data labeling
As artificial intelligence and machine learning continue to advance, the role of data labeling is likely to evolve. Here are a few trends and predictions for the future of data labeling:
Increased automation
As machine learning algorithms become more sophisticated, it is likely that data labeling will become more automated, with less reliance on human annotators. This could lead to more efficient and cost-effective data labeling processes, but it may also have implications for the job market.
Greater emphasis on quality
While automation may reduce the need for human annotators, there will still be a need for quality control and oversight to ensure that the data is accurately labeled. This may involve using machine learning algorithms to verify the accuracy of the labels or having multiple annotators label the same data and comparing their results.
More diverse data sets
As machine learning and AI are applied to a wider range of industries and applications, the data sets used for training will become increasingly diverse. This will require more flexible and adaptable data labeling approaches that can handle a wide variety of data types and formats.
Ethical considerations
As machine learning and AI become more prevalent, it will be important to continue to consider the ethical implications of data labeling, including issues related to data privacy and fairness.
Overall, the future of data labeling is likely to involve a combination of automation and human oversight, with a focus on quality and ethical considerations. As machine learning and AI continue to advance, data labeling will play a crucial role in ensuring the accuracy and effectiveness of these technologies.
Are you ready to take your business to the next level with the power of AI? Look no further than Tooliqa!
Our team of experts is dedicated to helping businesses like yours simplify and automate their processes through the use of AI, computer vision, deep learning, and top-notch product design UX/UI.
We have the knowledge and experience to guide you in using these cutting-edge technologies to drive process improvement and increase efficiency.
Let us help you unlock the full potential of AI – reach out to us at business@tooli.qa and take the first step towards a brighter future for your company.